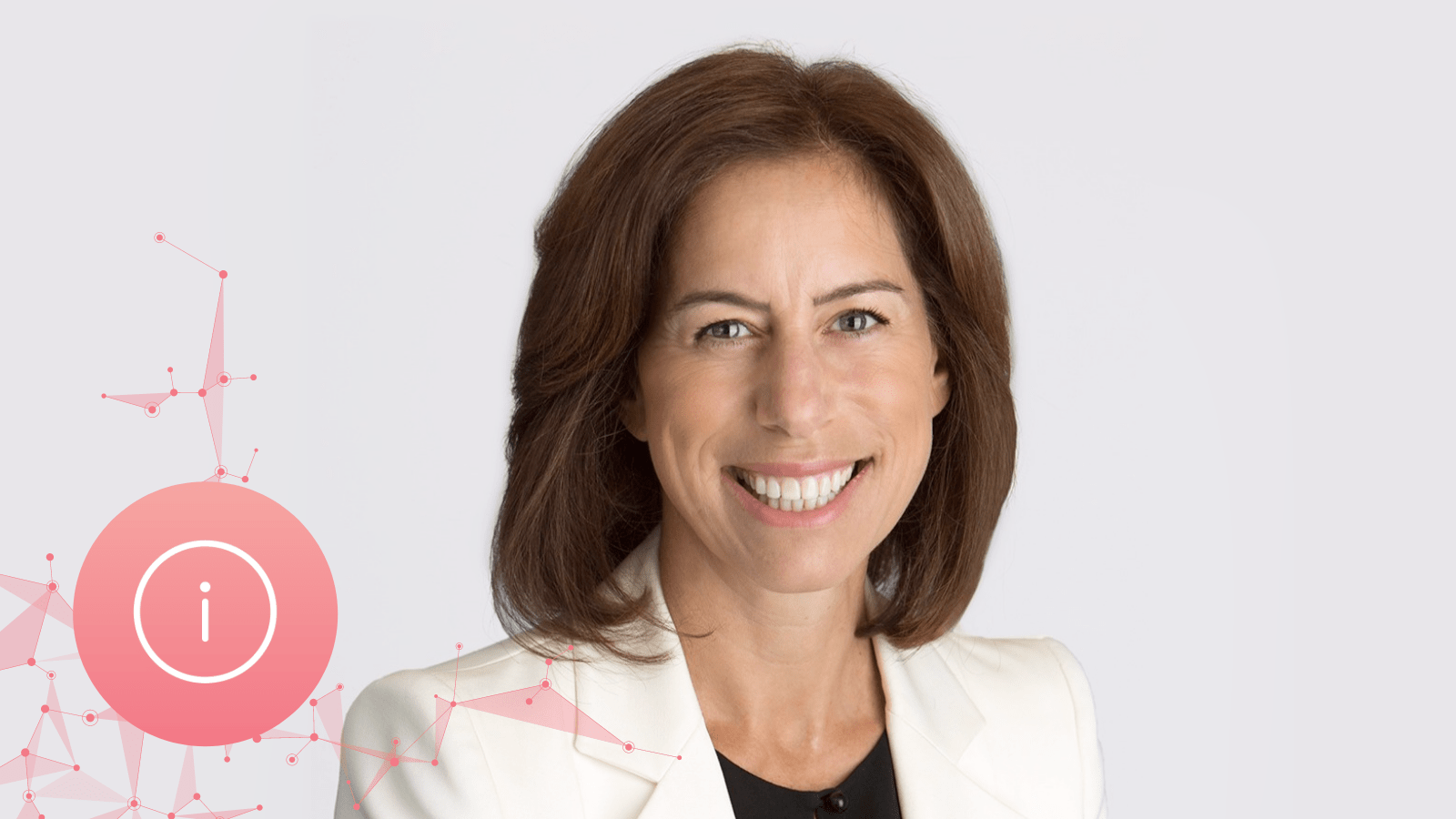
In this post, we explore the concept of ‘explainability’ with Jodie Wallis, Managing Director for Artificial Intelligence at Accenture.
The views expressed in this article are those of the interviewee and do not necessarily reflect the position of RBC or RBC Borealis.
Why is explainability central to ethical AI?
Jodie Wallis (JW):
Very simply put, explainability is about being able to detail how the AI came to the decision that it did in a given scenario, and what the drivers were behind that decision. Being able to explain how decisions are being made has always been important. But as the algorithms become more sophisticated and as AI starts to reach deeper and deeper into our decision-making processes, the need for explainability has become much more acute.
Do all algorithmic processes need to be explained?
JW:
No. And that’s an important distinction. Explainability really comes in when we are using AI to make decisions or recommendations that affect people’s lives in some material way. If an algorithm is being used to make a credit decision on a customer, for example, or to decide who to hire or promote – that is a decision that will require explainability. But if I’m using AI and a recommendation engine to decide which pair of shoes to offer you in an online store, I don’t believe that kind of algorithm necessarily needs explaining.
Why are organizations struggling with explainability?
JW:
I think one of the issues with explainability in AI is that it feels overwhelming and limiting at the same time. Many execs and IT leaders worry about the complexity and overhead they will need to create if they must explain all of their new models to numerous stakeholders before launching.
The problem with explainability is that the ease or difficulty with which you produce an explanation varies greatly with the type of algorithm you are using. The deeper the algorithm, the more difficult explainability is; the shallower the algorithm, the easier explainability becomes. And I think this has led some organizations to shy away from using certain types of deep learning algorithms.
How can organizations reduce the complexity of explainability?
JW:
It all starts with understanding which decisions and algorithms need to be explained and which do not. Right from the outset of the research, you need to know how important explainability is to the issue you are addressing. Does the action taken have a material impact on the life of an individual or individuals? If it’s not important, then the researcher or developer is free to explore any and all algorithms that might best fit their problem. But if explainability is going to be important, you will likely be limited in the types of algorithms you can use to solve that problem.
When we work with clients, that is almost always our first step – creating a framework to help decision-makers understand which actions require explainability and which do not.
Are there any tools to help simplify the explainability process?
JW:
No. And, frankly, I think the market is currently very immature in terms of the technical tools to help manage these aspects of responsible AI.
There are a few different schools of thought as to how you do explainability of deep algorithms. Some researchers and scientists are using reverse engineering techniques where they study the outputs and patterns of a sophisticated deep learning algorithm in order to create a less sophisticated model that is able to simulate those outputs in a more explainable way. The problem is that they are trading off a certain amount of accuracy in order to achieve explainability. But in some circumstances, that may be a worthwhile trade-off to make.
Ultimately, every situation will be different and there are no tools that truly ‘solve’ the explainability challenge. That’s why it is so important that designers and developers understand the need for explainability at the very start of the project – at the point where they can build it into the design.
What role will regulation play in supporting explainability?
JW:
I think governments and privacy commissioners will need play a key role in this area. Some are already making inroads. In Europe, for example, the General Data Protection Regulation (GDPR) talks about a person’s right to “meaningful information about the logic” when automated decisions are being made about them. Individual regulators are also looking at the challenge – Singapore’s monetary authority, for example, has published guidelines around explainability. But, currently, regulation is still pretty nascent.
What can designers and developers do to help improve explainability?
JW:
This is about putting explainability at the very start of the process. Before you go and start solving for a particular business problem, you really need to understand the ultimate need for explainability. There’s no use developing a cool and sophisticated new tool if the business is unable to use it because they can’t explain it to stakeholders. So it is critical that developers and designers understand what will require explaining and select their tools accordingly.
What can the business community do to improve AI explainability?
JW:
I believe business leaders recognize that explainability is one element of their responsible AI strategy and framework. If they are not already thinking about this, I would suggest the business community spend a bit of time creating smart policies around the explainability of algorithms and extending existing frameworks – like their Code of Business Ethics – into AI development.
That will lead to two key value drivers for businesses. The first is that organizations will be freer to develop really interesting value through AI solutions. But, at the same time, they will be contributing to the societal discourse around the need for explainability. And, given the growing importance of the topic to consumers, regulators and oversight authorities, that can only be a good thing.
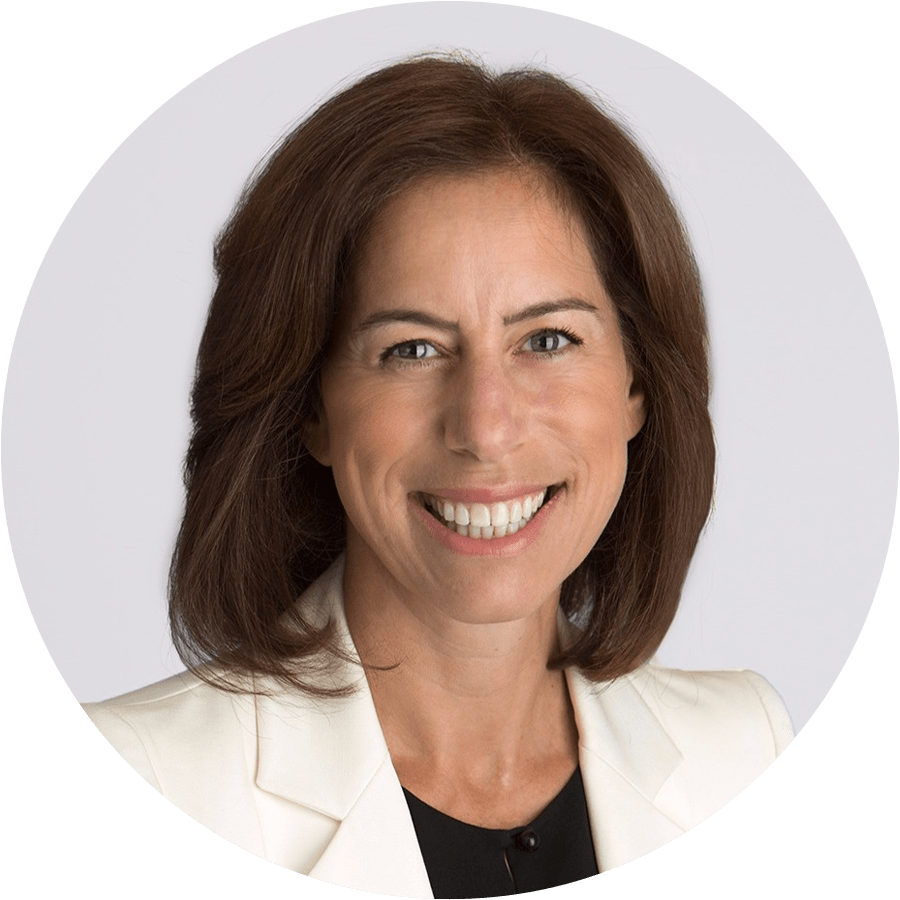
About Jodie Wallis
Jodie Wallis is the managing director for Artificial Intelligence (AI) in Canada at Accenture. In her role, Jodie works with clients across all of Canada’s industries to develop AI strategies, discover sources of value and implement AI solutions. She also leads Accenture’s collaboration with business partners, government and academia and oversees Accenture’s investments in the Canadian AI ecosystem.
News