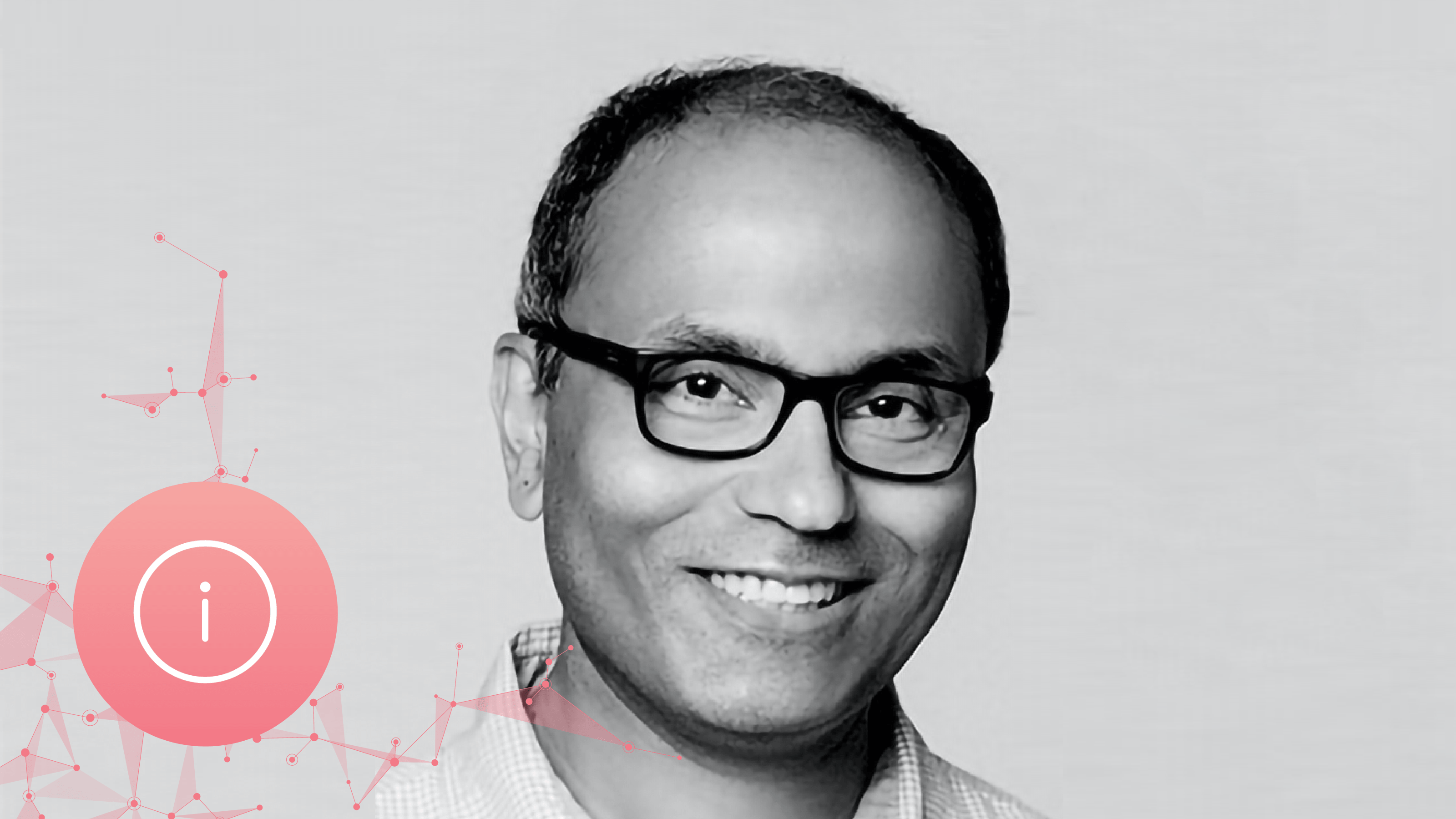
In this interview, we talk to Prasad Chalasani, CEO and Co-Founder of XaiPient, an AI startup that building focuses on products empowering analysts to make better business decisions with no-code trustworthy AI.
The views expressed in this article are those of the interviewee and do not necessarily reflect the position of RBC or RBC Borealis.
What does XaiPient do?
Prasad Chalasani (PC):
Our focus is on enabling business analysts to use the automated ML and explainability to make better decisions. What we found is that there is a world of non-coder business analysts out there who want to use business data and machine learning to deliver actionable predictions. But they also want to understand the drivers behind those predictions, so they can trust and act on them. Our solutions are aimed at helping them achieve those goals quickly, getting insights they need in minutes, rather than waiting for days or even weeks for data scientists to build ML models.
Would data scientists and ML engineers find your solutions useful?
(PC):
Certainly. And we also offer a model training and explanation API for data scientists and ML engineers. But we have found that data scientists are generally quite comfortable using open source explainability tools that enable model developers to show factors influencing predictions. Business analysts, on the other hand, can’t adapt tools to their business contexts as easily, since these adjustments would require ML modeling skills, and tend to be more interested in the drivers behind the predictions that influence business outcomes. That means their capabilities and needs are somewhat different.
How has your business model evolved as you explored the market need?
(PC):
We have learned a lot over the past year and half. Initially, our plan was to bring our solutions to technical teams as a pure explainability tool. But we quickly realized we needed to pivot. Now we are getting lots of traction in the market by packaging AutoML with explainability tools focused on non-technical teams. The conversation isn’t about explaining their models; it’s about understanding their data and improving the business.
What are some of the main use cases you’re focused on?
(PC):
Real-world applications have been very exciting. We recently used our event-sequence models approach to identify which government actions were having the greatest impact on COVID-19 death rates. We can potentially also use these models to figure out if a certain type of drug or therapy leads to an increase in adverse events.
At the other end of the spectrum, we work with clients to drive marketing analytics. For example, we show which accounts are most likely to convert, and help identify campaigns and channels with the most impact on predicted conversion rates. This helps businesses see the real drivers of their business, faster. These drivers can then be adjusted to transform the business and improve the outcomes.
You recently co-authored a paper that suggested a link between adversarial learning and explainability. Can you summarize your findings?
(PC):
For that research, we focused on the following two desirable properties of feature attributions: sparsity and stability. Sparsity means making the attributions human-friendly by focusing on only the truly relevant features. Stability ensures that you don’t get wildly different explanations if the input changes slightly.
It turns out that, when you train a model to be robust against adversarial perturbations, you also tend to get sparsity and stability as side effects. If you picture the adversary strength as a knob you can adjust, you want to find that point where attributions become sparse without impacting the natural accuracy of the model. When it comes to stability, we found that training an ML model with an extra regularizer penalizing instability is actually equivalent to adversarial training.
What are your thoughts on the levels of accuracy and trade-offs between accuracy and relative probability?
(PC):
In some domains, such as adverse drug event prediction, the precise probability is extremely important. But in the marketing use case, for example, it’s the relative predictions that are the key. When the marketing team provides the sales team with a list of accounts that, based on their models, are most likely to convert, the sales team isn’t worried about the exact probability of conversion but rather the relative probability versus other customers.
How do you expect the field to evolve?
(PC):
I believe the opportunity for these types of augmented analytics solutions is massive. I think there is significant demand amongst the business analyst community for solutions that can augment their current business intelligence tools with AutoML that come with the rationale built-in. We have a general-purpose business intelligence engine called XBI and a specialized engine focused on the marketing use case. But we see growing demand for similar tools across the business analyst space.
We’ve also been putting a lot of focus into asynchronous event sequence data which we believe is being largely ignored in the open source solutions for AutoML and explainability. It’s one thing to take nice looking tabular data and generate explanations; it’s another thing to work with messier data sets like event sequence data. I suspect that’s where the innovation is going to come from in the near future. One of our ICML 2020 papers proposes a flexible model for such data, and shows a way to infer causality among different types of events.
Do you have any advice for those looking to commercialize their ideas in the machine learning space?
(PC):
I think it’s a golden time for startups in this space. Many great tools are being developed in the data-engineering and ML ecosystem. Machine learning is still a hot topic and there is lots of investor interest in new companies in this area.
If I had a word of caution, however, it would be to think carefully about the plan for commercialization. When you have an idea, it’s very tempting to get to work building things. But, as we learned, you really need to think carefully about the commercialization. It took us some time to understand how our idea created value for businesses. Being thoughtful about the go-to-market strategy can save valuable time wasted talking to the wrong audience.
Where is your business going from here?
(PC):
We’ve been exploring some exciting partnerships with different marketing analytics and Customer Data Platforms. And we are currently running a number of private beta tests with customers using our XBI engine. In the longer term, we are focused on pushing these products out to the market, expanding our team, creating new partnerships and building new solutions. It’s going to be an exciting time.
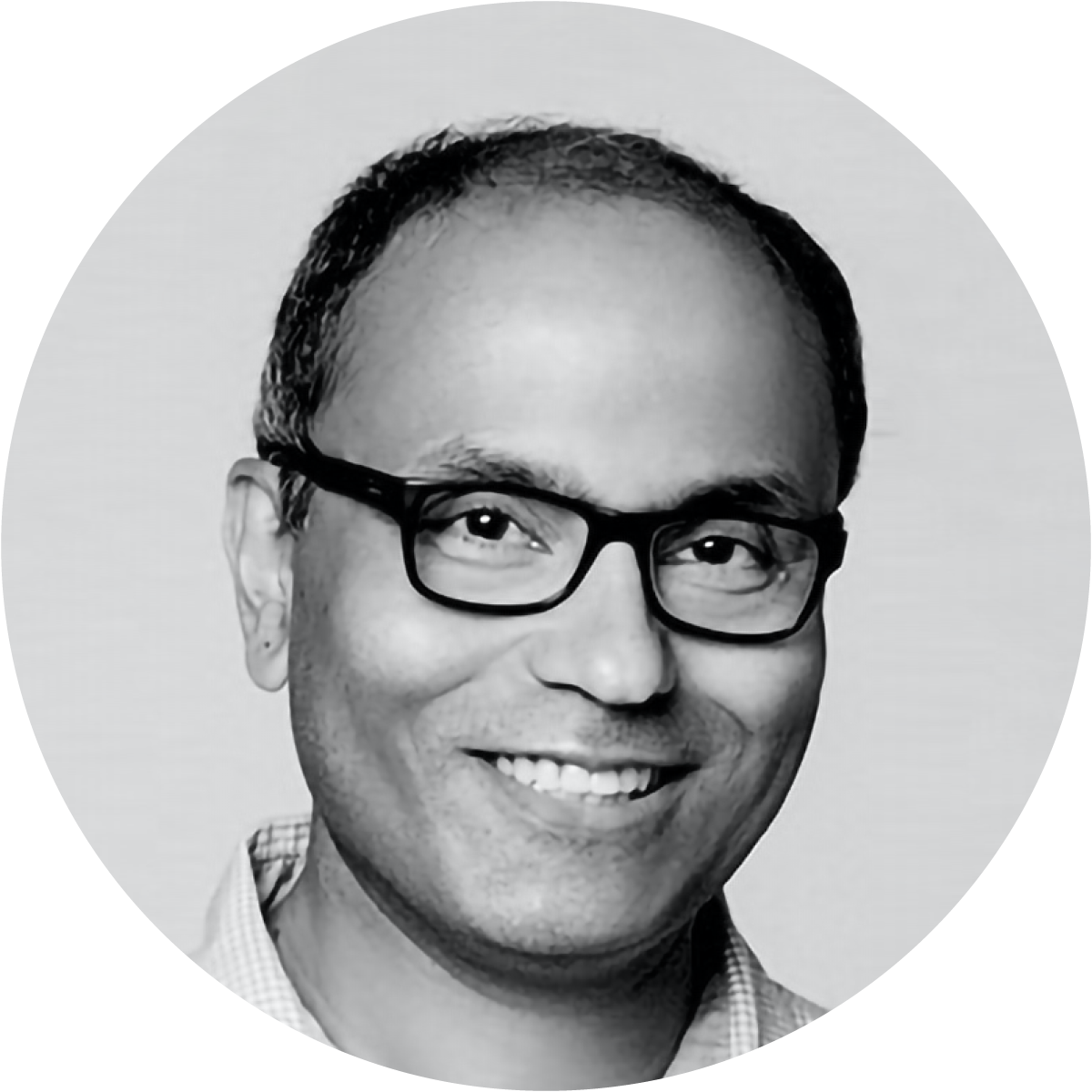
About Prasad Chalasani
Prasad Chalasani is the Co-Founder and CEO of XaiPient, a New-York based AI explainability start up. With 20 years of experience leading quant and ML teams at some of the world’s leading organizations – including Goldman Sachs, Yahoo and MediaMath – Prasad is active in the field of trustworthy AI research and has published several papers in the field of machine learning.
News
Field Guide to Machine Learning Product Development: What Product Managers Need to Know
News
How to navigate the model and product development phase of your machine learning project
News