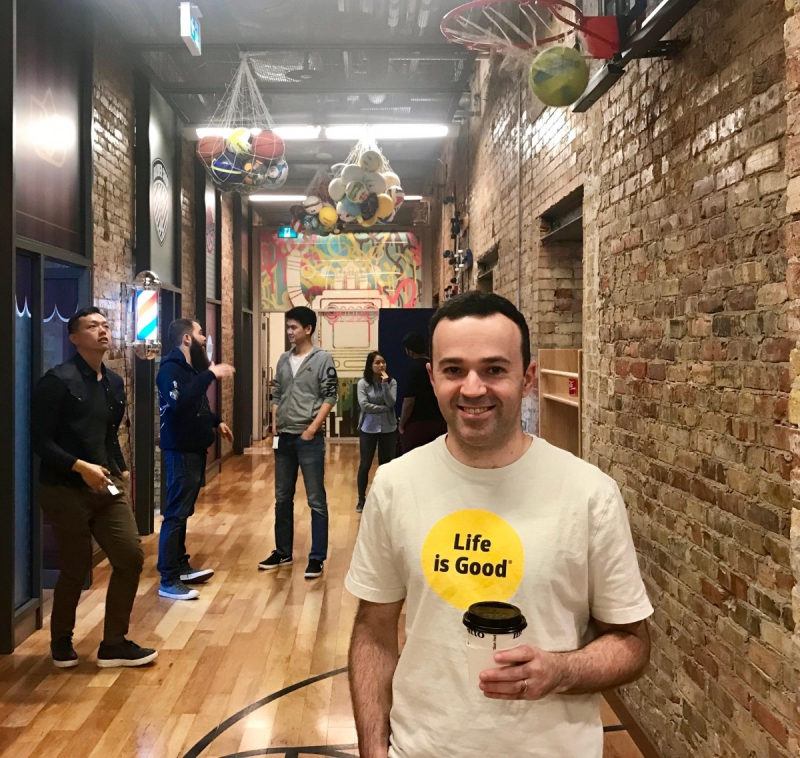
Life is good for our applied research team and not just because there is literally a guy in the picture wearing a shirt that says, “Life is Good” and they took it as a corporate directive. The two things are linked, however. That’s because the guy wearing the shirt is Yevgeniy Vahlis, and he’s our new Director of Applied Machine Learning.
Yevgeniy’s presence in our lab means many things. It means the researchers and research developers focused on the business side of our adventures in NLP, unsupervised learning, reinforcement learning and graphical modeling now have a fearless (and brilliant) leader to help guide their work. It also means we have that rare creature – an accomplished academic with strong business and management experience and a background in machine learning – to help take the incredible momentum we’re building to levels beyond.
Get to know what makes Yevgeniy tick, why he has a thing for keys (but not those kind of keys), and what his vision is for the Applied Machine Learning team beyond sparring with Cathal Smyth for t-shirt supremacy (pictures supplied on demand).
Before we talk about how you ended up heading an applied machine learning research team, what were you like as a kid?
I was a pretty nerdy kid. I used to play with electronics a lot. I know it sounds cliché but that’s the reality. Some people were really sporty when they were kids, then they got into tech. I was always a nerd. My friends were also nerds… I ended up going into computer science at university.
Was that an obvious choice?
[No answer].
Got it. OK.
[Acting like this brief interlude never happened] So then I came to Canada – at University of Toronto – to do grad school. The field I was interested in at the time was cryptography, which is the theory of encryption: how you protect information, not through providing access to it, but by actually giving it to someone and having them unable to tell what that information is without a key.
What drew you to that field?
What appealed to me in crypto is that it’s really hard math and you can’t cheat. I like the challenge, the difficulty of the problem. It’s similar to machine learning in some areas because you can’t fake it: if it doesn’t work people can break it. So, the solutions have to work, otherwise you’re screwed.
What’s one of the most common misconceptions about cryptography?
The most common misconception, and it’s so prevalent, is that people think if they personally can’t see a way to break something, that it’s impossible to break. There’s a saying in cryptography, “Everyone can build a system that they themselves cannot break.” If you’re the only one trying to break something, you will inevitably find a system you fail to break. There’s a very strong intuitive pull to think it’s unbreakable. It’s how people think, but it’s not true.
Does this have a natural application to cybersecurity?
They’re both security topics, but they’re completely unrelated – and that’s another misconception about cryptography. Cybersecurity is more about systems, networks, how do you make your code correct to prevent someone from getting to the problems that exist in the lower levels of code you don’t know about. So, it’s a lot more about engineering than science. Most of cybersecurity is about I have this massive system that has millions of components from various sources that behave unexpectedly. How do I prevent someone who knows how to exploit that from getting to the point where they can exploit it?
How does your broad experience in cryptography lead you to heading an applied research team in machine learning?
It’s not a one-step journey. I was working as a cryptography researcher at AT&T Labs in New York, which is also where I met my wife. We decided New York was fun but not where we want to build our family, so we came back to Canada in 2014. But back then there was no research happening in machine learning outside the universities – nothing like RBC Borealis. There was some basic data science, but no science science. I decided to go into industry and it was my first step into non-research. I did engineering, machine learning, security, everything. I worked at Amazon in their demand forecasting group building large-scale machine learning systems, which was a fascinating experience. I learned a lot on the business side and how to execute. From there I moved to a VC fund called Georgian Partners, where I used my expertise and experience to help the start-ups Georgian invests in on the product and technology side. I completed several machine learning research projects with the start-ups and they loved it. It was like being part of their team and it made a real impact.
Sounds like a great gig. What made you decide to walk away from that experience and come to RBC Borealis?
The main thing is Foteini. I’ve known her for some time and I really liked working with her at Nymi. But the biggest thing is I think she’s honest about her vision. When you’re making decisions it’s critical you can trust the people you can work for. The other thing is, this is going to be a world-class AI lab. This kind of opportunity doesn’t crop up more than once in a lifetime. Borealis is different from other labs in that we’re defining things as we go. There are labs whose objective is just to publish and there are applied teams that have very specific business-driven goals. We’re defining both and I think this is the only place that has real research and is not yet fully constrained by the business.
What’s your vision for your team?
I want the team to be happy and to feel they’re accomplishing something big. The way to do it is to find these problems I just talked about – a few things we think are solvable through significant effort – and if we solve them we will literally change the way the bank works. The [fundamental] theory team is pushing the state-of-the-art in machine learning; [the applied team is] going to be transforming the bank. We’re a small team; the bank is massive. The vision is that we can still do this despite our size. But I’m also excited about taking our work beyond the bank and applying it to make changes in society, helping NGOs, charities. It’s pretty encouraging that we have access to these types of resources, including the brain power in the room. It’s up to us to lose this chance. It’s ready for us here, we just need to use it appropriately. I’m excited about this direction.
One of the biggest challenges facing AI as a field is figuring out the nuances and the structure building up around an industry that’s still defining itself. This is particularly difficult for anyone in a management role. What have you found to be particularly tricky?
There’s the research mindset which is, “I need a hard problem to solve.” There are a lot of problems like that. Not all of them are worth solving. How do we find the right things to go all-in on which will satisfy the need for these hard problems, but at the same time will actually change the way things are done? I know there are problems that if we applied this brain power to them, things will change a lot. I’m hoping to really focus the applied team on problems like that. We already have a research team, so it’s about finding the right problems to spend our time on.
Is there a risk that in the race to achieve state-of-the-art, we’re missing some crucial steps or perhaps pushing things in the wrong direction just to be first instead of slowing down to make sure we do AI right?
In science, either you’re right or wrong. It either works or doesn’t. There may be things that work better, but you’re just trying to push things forward as fast as possible. Except even when you do, you’ve pushed things forward that didn’t exist before. You’ve made progress. It’s irreversible. Maybe if we took a step back and looked closer without rushing we could have made a much bigger step, but that’s human nature, and perhaps wanting to be first is what drives our progress. So, I’m not too worried about that.
Ultimately, I don’t think we have a choice. We can’t completely ignore the market, or we’ll get driven out of existence and you’ll only see results every few years. But there’s room for both fast and more thoughtful types of approaches. There are people who think research should be long-term even in a capitalist environment, but once you have a free market, things fall into place on their own. People do what they need to do to stay relevant. Within a small environment like this, however, we can decide what’s long- or short-term and we can do both.
News
Introducing RBC Borealis
News
RBC Borealis to open new artificial intelligence lab in Montreal McGill professor Jackie Cheung to act as academic advisor
News