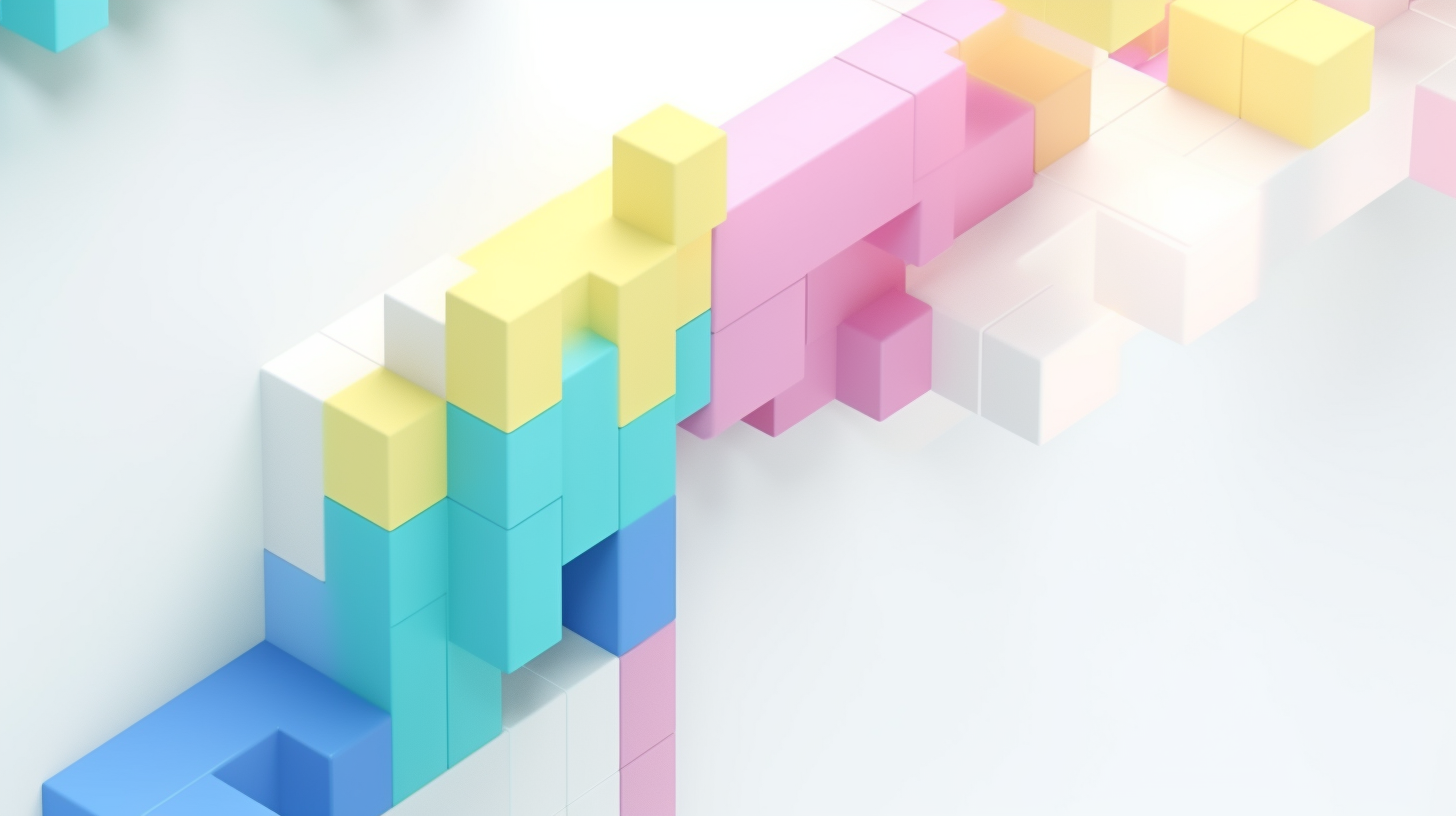
Companies are looking to deploy AI in a way that keeps them competitive while also building trust with their stakeholders and communities. In this article, we talk with Preeti Shivpuri, a Director in Deloitte’s Data and Analytics practice, about the challenges faced by clients as they work to build sound AI governance and Trustworthy AI practices and the advice she is offering them.
The views expressed in this article are those of the interviewee and do not necessarily reflect the position of RBC or RBC Borealis.
Why is responsible and trustworthy AI moving up the corporate agenda?
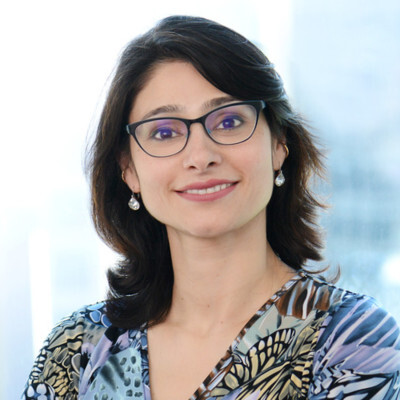
Trustworthy AI is about "responsible competitiveness", focusing on reinforcing trust and confidence with customers, shareholders, regulators and partners within the ecosystem. And that means incorporating ethical principles into AI development and deployment.
Organizations want to leverage AI or business innovation and are collecting all types of sensitive and personal customer data. But just because you have and can do something with data doesn’t mean you should do it. The risks, liabilities and negative consequences from AI systems (such as fairness and transparency risks) are being recognized as a Board level agenda and heightening the reputational risk to the organization. As customers become more conscious of ethical considerations, they are more likely to choose companies that demonstrate responsible AI practices. Also, this is not a one-time activity. We believe it is something organizations should be doing every day and with every decision.
At the end of the day, trustworthy AI is another way that organizations are able to demonstrate their values, beliefs and principles to their stakeholders and customers.
How can organizations reinforce trust with responsible AI?
Responsible AI or Trustworthy AI requires a holistic approach that is embedded throughout the AI development lifecycle and considers how the model will be operating within the ecosystem, keeping both internal and external stakeholders in mind.
Organizations should consider developing Trustworthy AI or AI Governance frameworks and principles that focus on the following:
- Transparency and Explainability
Users and stakeholders should be able to understand how AI algorithms make decisions and what data is being used. This transparency helps build trust and allows individuals to understand what went into the design and decision-making process. - Fair and Impartial
Taking deliberate steps to mitigate bias. Consider how data is trained and ensure systems are continuously monitored. Where possible, use DEI principles and human-centric design approaches when developing AI systems. - Privacy and Security
Following data privacy and data protection requirements helps ensure customer data is handled and protected in a responsible and compliant manner and that individuals’ privacy rights are respected. This also ensures that data is governed throughout its lifecycle, from collection through to disposal. - Accountability
This demonstrates that accountability and human oversight have been established and that organizations using, designing and implementing AI systems understand their role and can be held accountable. Auditing AI systems is also a way for organizations to demonstrate how seriously they take ethics and Trustworthy AI, and to reinforce trust. - Compliance with regulations
In the current times when regulations are still being shaped, organizations should be proactive in setting their own standards and adhering to them. A proactive approach to meeting regulatory expectations also enforces trust and demonstrates your organization’s values and principles.
What that means in practice is having a diverse set of stakeholders sitting at the table engaged as the systems are getting designed and developed. Not just legal and privacy but also third-party vendors and software providers and other partners in your ecosystem. These perspectives need to be included at the start, not as an afterthought.
How will regulation influence corporate activity in this area?
Regulatory stances are still taking shape, but we can see the current prime focus is on using customer data responsibly with data ethics in mind. With GDPR, the EU AI Act and even Quebec’s Bill-64, the focus is on data protection and informed consent.
Another key insight highlighted through the recent regulatory discussions is the need for a risk-based approach in designing and implementing AI testing or AI/ML validation processes within the AI development lifecycle. For example, the recent NYC Local Law 144 (regulating the use of automated employment decision tools (AEDTs)) explicitly requires organizations to go through independent Bias Audits.
Another one that is not a regulation but enforces good practices is the ISO/IEC 42001 standard currently being piloted by the ISO/IEC Committee for Artificial Intelligence. This emphasizes the rigour needed when using or designing AI systems and highlights that AI Management Standards will be integral to helping organizations successfully reinforce trust and provide assurance to customers on effective AI governance and oversight.
We hear from our clients and recognize that the challenge for most organizations today is just staying on top of these regulatory changes/standards and customizing the existing processes for their organization with the right balance in mind – governance embedded and paired with development approaches that do not stifle innovation, but ensure appropriate guardrails are being designed and implemented.
What type of risks should companies be looking for and how can they mitigate them?
When we talk about trustworthy AI, we tend to look at the risks across six main dimensions:
- Fair/Impartial
- Safe/Secure
- Responsible/accountable
- Transparent/explainable
- Robust/reliable
- Data privacy
Inherent to these is effective data governance and third-party management. Paired with these risks, we should also look at the context of where the model is going to be used, what algorithm or technique will be applied, and what data will be ingested to train the model.
It’s not going to be one common set of risks that runs across everything in the same way, and hence an AI risk impact assessment should be a critical step that organizations should design. Assessing the impacts of AI risk early on as part of the model development lifecycle with all stakeholders in mind is critical. At Deloitte, we have a tool that helps support early and continuous assessment of AI risks for each use case, taking into account industry standards and regulatory expectations. The tool provides AI developers with immediate actionable guidance and mitigation approaches to consider as part of their development process.
The real trick, however, is operationalizing it. And that’s where embedded workflows and automation come into play. It’s the clear workflows that allow the risk partners such as privacy, legal, compliance, security, model risk management teams and others to be engaged early on within the development process.
As such, organizations should consider developing a robust AI governance framework and embedding it within a standardized model development lifecycle (MDLC) process enabled by automation and workflows.
What advice are you offering your clients?
Key measures organizations can take as they embark on this journey are:
- AI Literacy programs
Organize sessions for business and tech leaders, data users and the Board to help them understand the responsible use of Data & AI, as well as to raise awareness of trends/practices and the ongoing regulatory/compliance evolution. You might build that capability yourself, or you might lean on a partner like Deloitte that runs AI Institutes and offers things like sandboxes where leaders and executives can have a safe space to explore the risks and what some of the mitigation measures might be.
An aspect to consider is your organizational culture, spanning not only the company’s operating culture but also its data culture. My experience suggests that – when a company really raises the bar on the data culture – that’s when change happens, and you start to see trustworthy data and AI as part of the Board agenda. - Initiate a Trustworthy AI or AI governance and policy program
Clearly articulate your definition of AI and how you expect it to be designed and implemented. Create ‘net new’ and, where applicable, enhance your existing policies (particularly related to third-party vendors, privacy, data and technology) to clearly highlight expectations and to set the tone for the organization to follow. Assess the evolving regulatory stance and ensure those are communicated and reflected within the policy, processes and implementation approaches. - Continuous monitoring and end-to-end MDLC process
The third thing I would suggest is to have an approach or strategy for monitoring and regularly assessing your AI models. We all know that models drift, particularly when they are learning at the speed they are today, so you really need to manage this upfront. Reflect on the impact this will have on existing processes, such as system development or developing the MDLC process, and ensure the right guardrails are implemented and reflected within those processes.
Are you optimistic about the relationship between humans and AI?
At Deloitte, we see the relationship between humans and AI as ‘The Age of With’. AI tools should be an enabler for everything we do, and they should be focused on allowing us to do better things in the global society we live in.
The opportunities we are uncovering today are limitless, and it is just the tip of the iceberg. But there are risks embedded in the current datasets (and mindset) which need careful and deliberate considerations in design and training. We need an approach that puts responsible and ethical considerations front and center. And that would deliver massive benefits for industry and the global and collective society we live in.
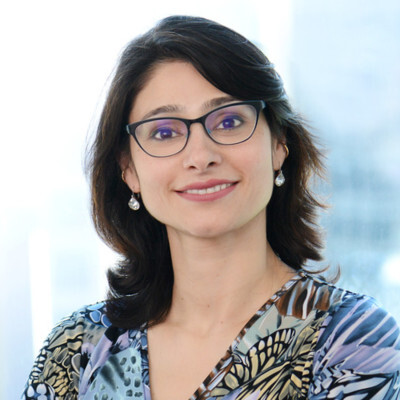
About Preeti Shivpuri
Preeti is a leader in data and analytics strategy, helping organizations effectively manage data and information assets to generate insights, drive growth and operational efficiency while meeting evolving regulatory demands. She advises clients on execution strategies and sound data and AI governance, enabling them to realize benefits aligned to their business goals throughout their data insights journey. She leads Trustworthy AI and Ethics within Deloitte, helping organizations to operationalize and scale AI solutions responsibly with the right balance of innovation vs controls.
Advancing Responsible AI
Responsible AI is key to the future of AI. We have launched RESPECT AI hub to share knowledge, algorithms, and tooling to help advance responsible AI.
Visited RESPECT AI hubNews