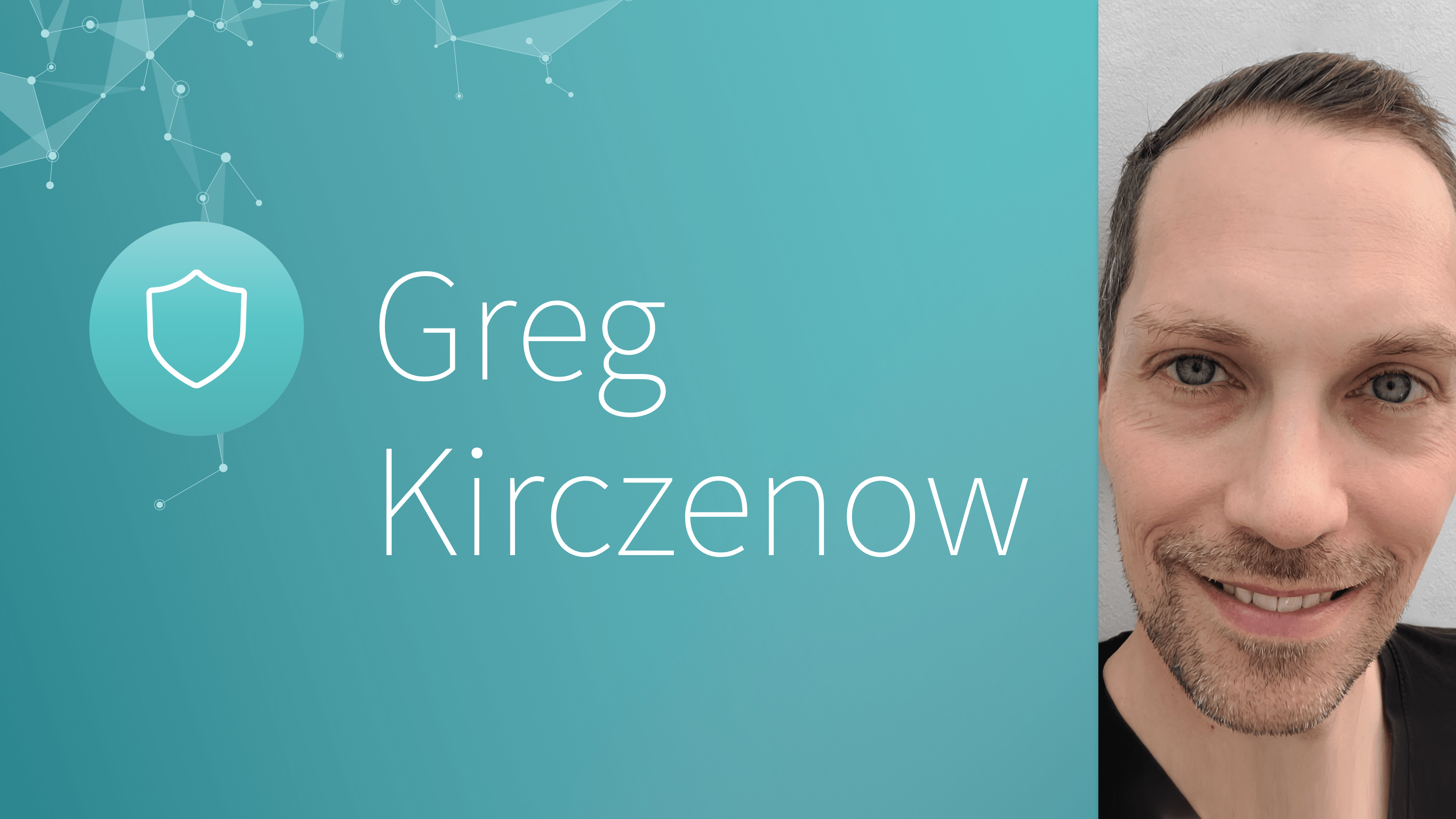
In this post, we explore the concept of ‘independent effective challenge’ with Greg Kirczenow, Senior Director of AI Model Risk Management at RBC.
The views expressed in this article are those of the interviewee and do not necessarily reflect the position of RBC or RBC Borealis.
What is an ‘independent effective challenge’ and why is it important?
Greg Kircznow (GK):
The idea is to create some really good checks against cognitive bias in models. At its most basic, an independent effective challenge is about motivating a group of experts to check the work of another group. That is often the best tool available for ensuring models are working correctly. And the more complex the model, the more value that comes from having another set of experienced eyes verifying that everything is working as expected.
Are models and their designers naturally biased?
GK:
I think it’s very difficult for people to see their own biases and to know how to mitigate them. So, if you are a model developer you might need a second set of eyes – that independent effective challenge – to see what you are not seeing and identify problems before they come up in production.
Is it difficult to find the right people to perform an independent effective challenge?
GK:
It has been a challenge. I think there is the perception that anyone with a statistics degree can quickly develop expertise in the various kinds of machine learning models. But my experience has been that it is a steep learning curve. When I’m hiring, I’m looking for people with a high level of expertise because their job is to challenge the developers and that means they must be at the same level as the developers to do that.
Does regulation drive the requirements for model validation in the financial services sector?
GK:
The financial industry has been thinking about model validation for a long, long time. So, too, have the regulators. I believe that the model risk regulations are already broad enough and robust enough to encompass AI. The challenge, however, is that these regulations are largely principles-based which means we need to think carefully about what validation techniques we can use to ensure we abide by those principles.
Can independent effective challenge be replaced by explainability?
GK:
No. Frankly, I think there is a popular misconception that explainability is a prerequisite for model robustness and model fairness. But I would argue that it’s not only not necessary, it’s also not sufficient for testing robustness or fairness. Simply put, I believe we can have complicated models that we can trust to be robust and fair, even if we don’t have an explainability technique for them. The reality is that there are lots of tests we can run to determine whether a model is robust and fair, and those tests do not rely on our ability to explain how the model arrived at that decision.
Do users of the outputs of the models expect explainability?
GK:
If we are talking about the technical view of explainability – being able to document how an algorithm arrived at a certain decision – then no. I don’t think most users really want to understand how these models work, any more than I want to understand how my mobile phone works. What users want to know is that there are people responsible for knowing the model is working; that there is a team of professionals monitoring it; and that, if they find problems, that they can fix the mistakes. Of course, there are special cases. Model developers and validators find explainability methods to be helpful tools, and people sometimes want to know if there is an action they could have taken that would have resulted in different model output. We consider these kinds of situations carefully.
How do you see current approaches to testing AI models evolving?
GK:
We’re starting to recognize some of the limitations of current testing approaches. Consider, for example, the common approach of using holdout data sets for testing. That approach only demonstrates that the model works against a similar set of data. I think there is a wider recognition that we need to go deeper than that to think about how your model will perform sometime down the road. There’s a lot of research going into this area at the moment; I have personally been following teams working on developing common-sense tests for Natural Language Processing models. The findings have been eye-opening.
Do you use external model validation software platforms to conduct your model validations?
GK:
Many tools are being developed by different companies right now. The problem is that – for the financial services industry at least – the approaches are not fit-for-purpose for what we do in the FS industry. We need something that is really tailored to our sector and our business. That is why we are so excited to be working with RBC Borealis to develop and apply novel new tests. Right now, we’re focused on developing adversarial testing approaches. But we see lots of room for collaboration across the ecosystem to improve the way AI models are tested and challenged.
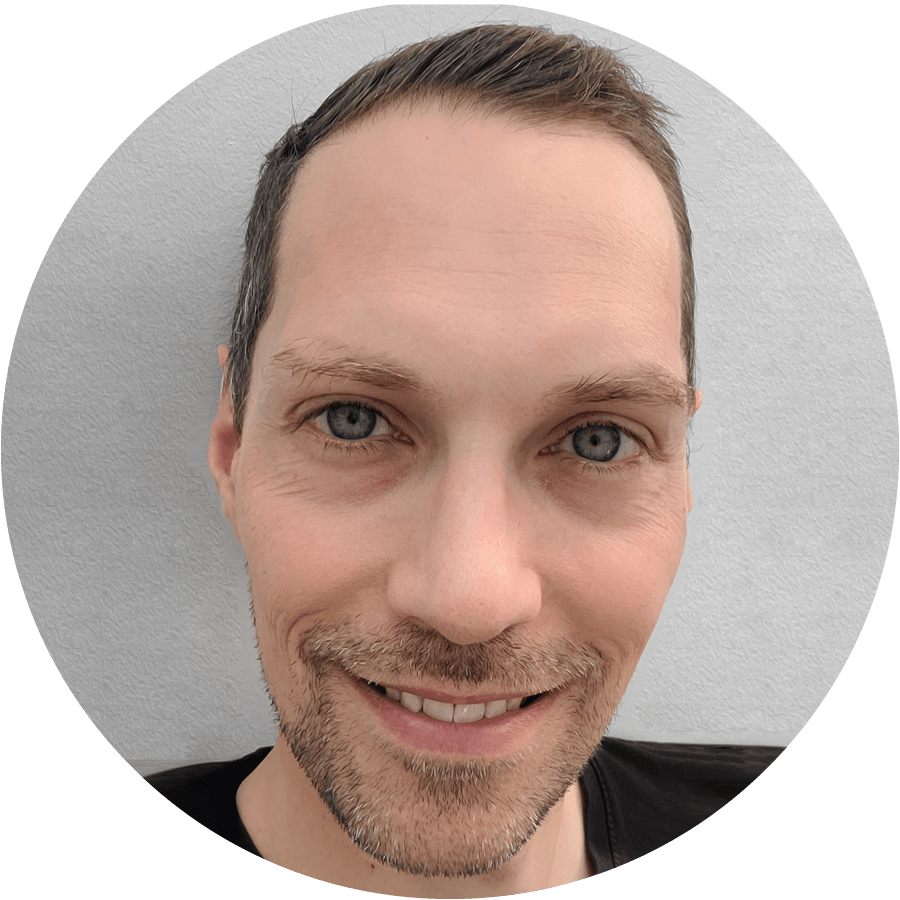
About Greg Kirczenow
Greg serves as RBC’s Senior Director of AI Model Risk Management. As such, Greg is responsible for developing and delivering the bank’s broad range of AI model risk frameworks, overseeing risk management activities and managing a team of independent and effective challengers.
News