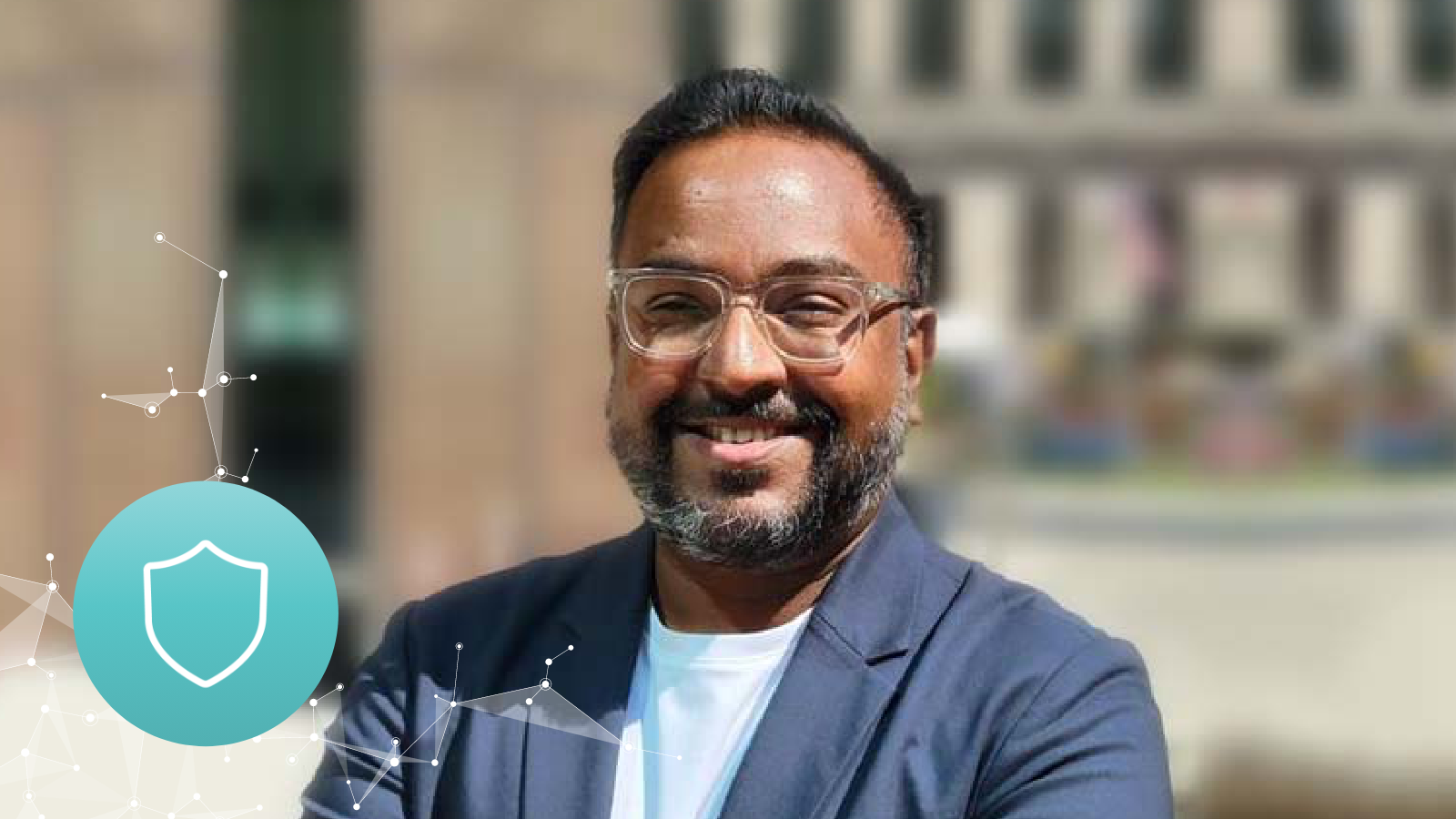
Businesses want to ensure their ML and AI models are making ethical, transparent decisions. In this post, Karthik Ramakrishnan, co-founder of Armilla AI, explains the importance of being able to govern, validate, test and monitor ML models to ensure they are responsible and trustworthy.
The views expressed in this article are those of the interviewee and do not necessarily reflect the position of RBC or RBC Borealis.
What is your go-to definition of Responsible AI?
Karthik Ramakrishnan (KR):
The broadest definition of Responsible AI is ensuring that the models do what we want them to do, and don’t do what we don’t want them to do. And by models, I don’t just mean AI and ML models; I mean any type of automated decision-making tool – from regression systems right the way through to neural networks.
Responsible AI means different things to different stakeholders. Take explainability, for example. It means very different things to your data scientists, your CEO, your Chief Risk Officer, your regulators, and the business team that owns the model. To the business, it’s more about justifiability – can you explain which features combined to trigger the outcome that transpired and justify the outcome in the context of the business decision. To the data scientist, it’s more about understanding which neuron fired at what point in the process. And that means it’s often easy to get caught up in the semantics.
At Armilla, we believe that Responsible AI isn’t a nebulous thing that we ‘eventually’ need to work out. It’s in the everyday practice of thinking about what you are building – thinking about how you ensure it does what it is supposed to do and doesn’t do what it isn’t supposed to do. That level of safety engineering practice needs to be brought in and ingrained into the DNA of the ML development team.
Everyone wants to de-risk AI and believes models should be safe for the end-user. But how well are ML and AI models tested and validated today?
KR:
The challenge is that machine learning and data science is still in its infancy. We’re still defining what responsibility means and what practices we can develop to ensure responsible AI. Data scientists do all sorts of testing, of course – unit testing, regression testing, integration testing and so on. Financial services firms will have groups of model validators to ensure their ML models conform to regulations like the E-23 Guidelines on Model Risk.
However, very few data scientists tend to undertake rigorous stress testing for business or regulatory requirements. In traditional software development, you have very established and mature practices around testing and QA. We need those types of established practices around ML, so we can ensure businesses and data scientists are building robust, transparent, and reliable systems. It’s a big gap that is missing in the industry’s practices.
What inspired you to start Armilla AI and how does your platform work?
KR:
We really came to the idea in a round-about way. It all started with work we were doing with the Monetary Authority of Singapore. Regulators wanted to understand how they could update existing regulation on model validation. We published a paper that outlined a principles-based approach identifying the types of things you should do when building an AI or ML model, and quickly realized we needed to get more granular, and so we then looked at each pillar in much more detail and offered suggestions on what likely tests would look like.
For the banks involved, however, the process was starting to add much more complexity. Risk and compliance teams were doing the testing manually. Production and development times were slowing down. Highly-material models could spend upwards of 6 months in validation. Seeing this first-hand made us realize it was something we could automate.
We bring together the various stakeholders at the beginning of the development process and work with them to specify what they want the model to do and what they don’t want it to do. And then we define those tests that allow us to measure statistically how the model behaves for these various conditions. As your team develops and iterates the model, they can run the various tests and the platform tells them which test cases are passing and which are failing. The platform goes into various scenarios to see how the model is behaving against the business criteria and the technical criteria, giving all of the different stakeholders visibility into how the model is doing.
As a result, everyone knows what data was used, how it was used, how it was tested, and so on, so that businesses can quickly move their models forward into development. It can be a complex set of processes, and we’ve made it a lot more efficient.
What type of data does your platform handle today, and how do you plan to evolve it?
KR:
We’re rapidly evolving the platform and in addition to structured time-sequence data models, we’ll soon have the ability to test vision-based and text-based models. NLP models are a bit more complex to test, but testing of text-based models will become even more important as more and more chat-bots and interactive models are developed. We also see demand for responsible AI spreading into other industries like manufacturing. Manufacturers want to know that the models they are developing for processes like QA are as accurate (or more accurate) than the manual status-quo. Establishing trust with the business around AI models is important in almost every sector. That’s why we’re expanding the types of models we deal with all the time.
What are the incentives for developers and product managers to improve their responsible AI efforts? Or will it come down to regulators requiring safe AI practices?
KR:
There is certainly lots of regulation in the pipeline. But I don’t believe we necessarily need regulation to solve this. What we need is more of the self-regulation that comes from teams who sit down and think hard about what kinds of issues could arise from that system, and then test for it with every version of that model. We’re not there yet. But I believe there will come a time where that kind of approach just becomes second nature in ML model development.
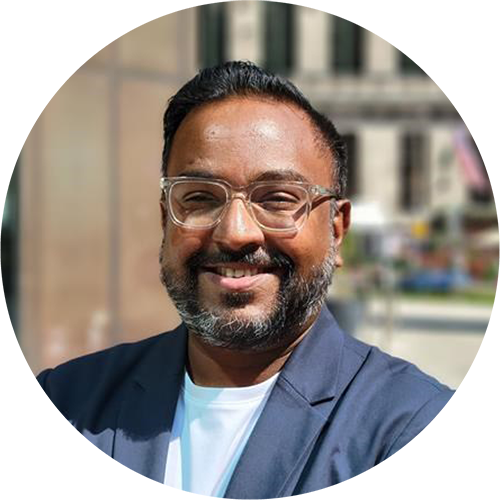
About Karthik Ramakrishnan
Karthik Ramakrishnan is the co-founder and Chief Product Officer at Armilla AI, based in Toronto, Canada. Prior to founding Armilla, Karthik headed up the Industry Solutions & Advisory activities at Element AI and led the Decision Science, Cognitive and IoT Analytics practice at Deloitte Canada. Karthik holds a Masters of Applied Science from the University of Waterloo and an MBA from the Ivey Business School at the University of Western Ontario. He and his co-founders also participated in the Y Combinator accelerator program in spring 2022.
News